Enhancing Machine Learning Success with an Image Annotation Tool
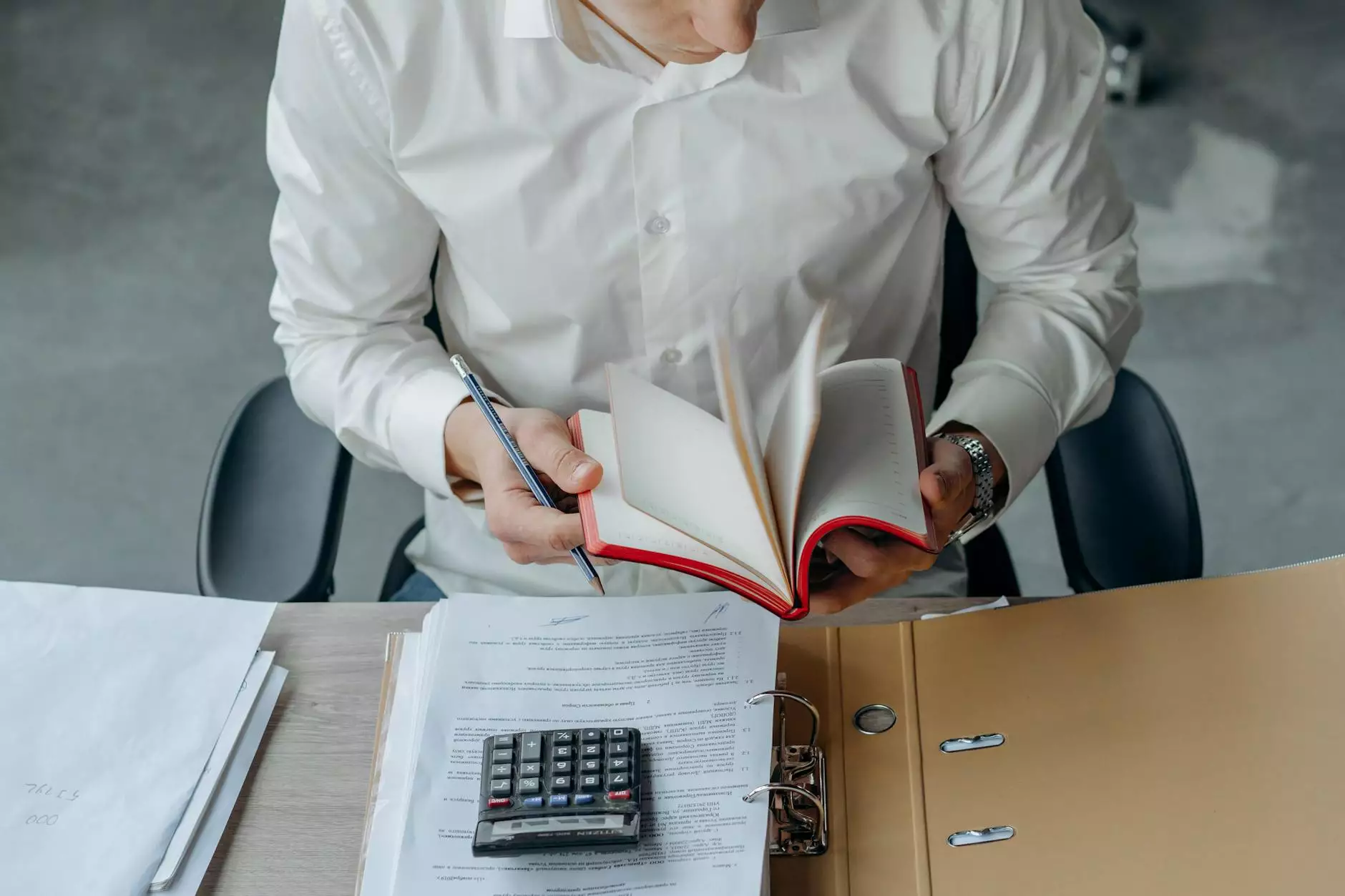
In the ever-evolving domain of machine learning, data quality is paramount. As algorithms rely heavily on training data, the significance of proper data annotation cannot be overstated. An effective image annotation tool is fundamental to achieving exceptional results in machine learning projects. This article explores how such tools empower businesses and enhance the efficiency of data annotation processes.
Understanding Image Annotation in Machine Learning
Image annotation is the process of labeling images with specific tags that define their content. This labeling is essential for training machine learning models, particularly in supervised learning, where algorithms learn from labeled data. The challenge often lies in the scale and complexity of this task, which is where an image annotation tool becomes invaluable.
Why Invest in a Reliable Image Annotation Tool?
Investing in a quality image annotation tool for machine learning can transform how businesses handle their image data. Here are several compelling reasons to consider:
- Efficiency: A good annotation tool speeds up the data labeling process through automation and intuitive interfaces.
- Accuracy: High-quality tools minimize human error by providing features like pre-labeling and validation checks.
- Scalability: Advanced tools are designed to handle large datasets, allowing businesses to scale their operations effortlessly.
- Collaboration: Many tools offer collaborative features that allow teams to work together in real-time, enhancing productivity.
- Integration: Supports seamless integration with machine learning frameworks and data pipelines, ensuring smooth workflows.
Key Features of a Top-notch Image Annotation Tool
When selecting an image annotation tool for machine learning, businesses should look for specific features that enhance usability and performance:
User-friendly Interface
A simple and intuitive interface significantly reduces the learning curve for new users, making it easier for teams to adopt the tool.
Variety of Annotation Types
Different projects require different annotation types, including:
- Bounding Boxes: Typically used for object detection tasks.
- Polygonal Annotation: Used for finer detail in defining object shapes.
- Semantic Segmentation: Labels each pixel specifically for tasks like scene understanding.
- Keypoint Annotation: Useful for applications like facial recognition where specific points on the face are important.
Quality Control Mechanisms
Tools that incorporate quality assurance steps, such as reviews and feedback loops, are crucial for maintaining high datasets’ integrity.
Customizable Workflows
Flexible workflows can cater to the specific needs of various projects, enabling teams to tailor their processes for better efficiency.
The Role of Data Annotation Platform in Machine Learning
A data annotation platform goes beyond just providing tools for image labeling; it encapsulates a whole ecosystem that supports various aspects of the data lifecycle. These platforms allow for:
- Centralized Management: Have a single hub for all data labeling tasks, making project management seamless.
- Team Collaboration: Real-time collaboration features invite input from multiple team members, enhancing creativity and accuracy.
- Access to Expertise: Many platforms have built-in access to a pool of expert labelers, ensuring high-quality output.
- Scalable Solutions: As businesses grow, their need for data annotation grows. A platform can adjust quickly to increased demand.
Best Practices for Using Image Annotation Tools
To maximize the benefits derived from using an image annotation tool, businesses should follow these best practices:
Define Clear Labeling Guidelines
Establish comprehensive guidelines for annotators to ensure consistency across the dataset.
Regularly Review Annotation Quality
Implement regular quality checks to catch errors and maintain the integrity of training datasets.
Leverage Automation Where Possible
Utilize automation features to handle repetitive tasks, allowing human annotators to focus on more complex labels.
Continuously Train Annotators
Invest time in training your teams to follow best practices and stay updated with advancements in annotation techniques.
Challenges in Image Annotation and How to Overcome Them
Even with the best tools, businesses can face challenges during the annotation process. Recognizing these obstacles can help in finding effective solutions:
Data Privacy Concerns
As businesses deal with sensitive images, ensuring data privacy is crucial. Utilize tools that comply with data protection regulations like GDPR.
Consistency in Labeling
Variability in how different annotators perceive the same image can lead to inconsistencies. This is where detailed annotation guidelines come into play.
Scalability Issues
Handling large volumes of data can overwhelm resources. Investing in robust platforms that offer scalability options is essential.
Time Constraints
Projects often face tight deadlines. Efficient project management and prioritization of tasks can alleviate time constraints.
Conclusion: The Future of Image Annotation in Machine Learning
As machine learning continues to penetrate various sectors, the demand for effective data annotation will only escalate. Businesses that embrace innovative image annotation tools and platforms will undoubtedly have a competitive edge in the rapidly evolving tech landscape. By focusing on quality data and leveraging the right tools, organizations can significantly amplify the performance of their machine learning models.
Take the Next Step with Keylabs.ai
If you're ready to elevate your machine learning projects, consider exploring the solutions provided by Keylabs.ai. Their advanced data annotation tool and comprehensive data annotation platform are designed to enhance efficiency and accuracy in your data labeling processes, ensuring your machine learning models are built on a strong foundation of quality data.
image annotation tool machine learning