Control System Machine Learning: Enhancing Business Operations
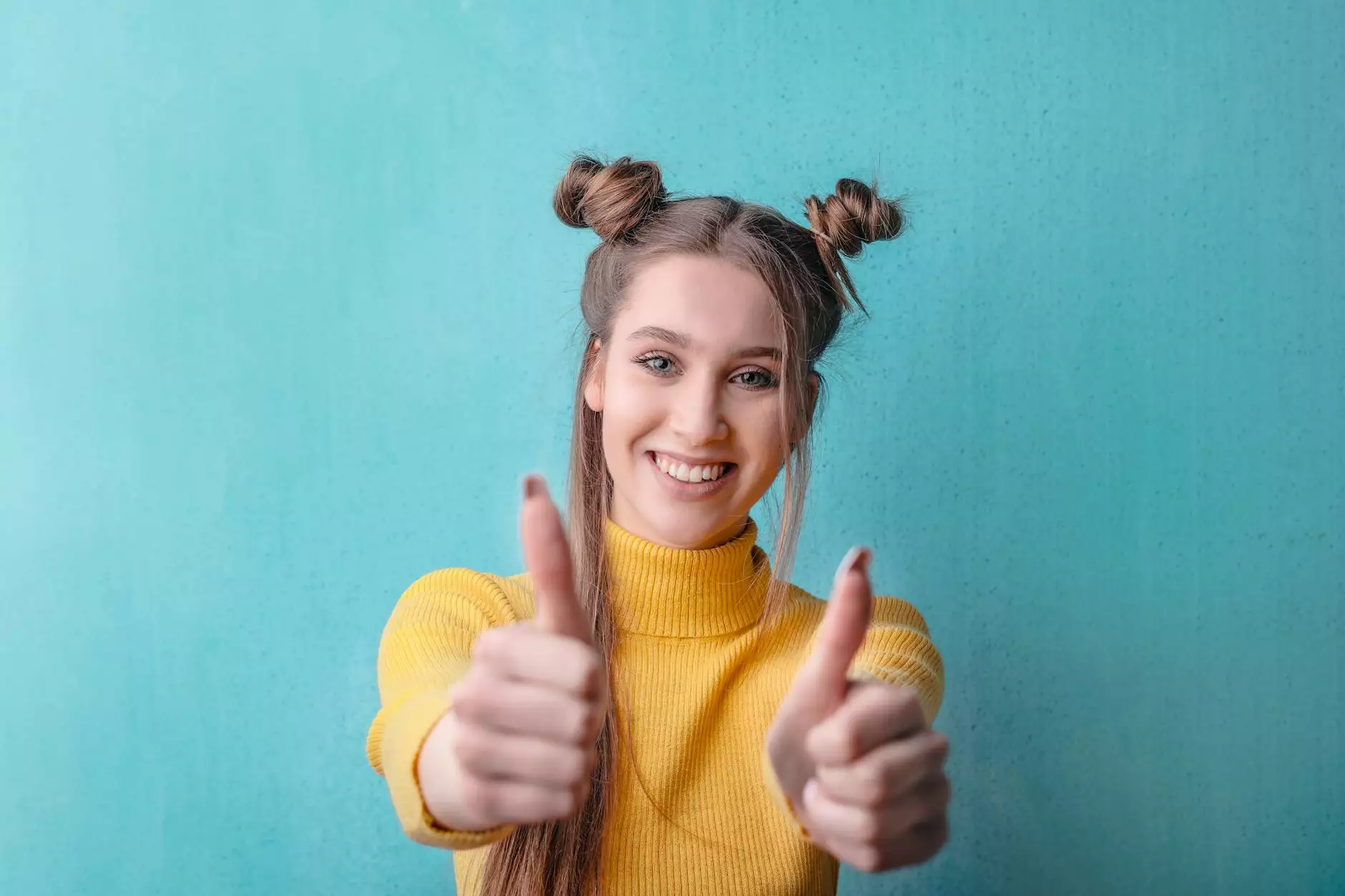
Introduction to Control System Machine Learning
In the rapidly evolving world of technology, control system machine learning stands at the forefront of innovations that redefine how businesses operate. Leveraging the potential of machine learning in control systems enables companies to enhance decision-making processes, automate repetitive tasks, and significantly improve operational efficiency.
This article delves into the significance of control system machine learning and its impact on various business domains, including content management services, business process automation services, and data governance systems. We will explore the functionalities, advantages, and practical applications of these cutting-edge technologies in modern business settings.
Understanding Machine Learning and Control Systems
Machine learning is a branch of artificial intelligence (AI) focused on building systems that can learn from and make predictions based on data. This technology relies on algorithms and statistical models to analyze patterns, automate actions, and make data-driven decisions. On the other hand, control systems are designed to regulate the behavior of various processes or systems, ensuring desired outputs regardless of external conditions.
When integrated together, control system machine learning combines these technologies to produce smart systems capable of:
- Enhanced predictive analytics: Anticipating future outcomes based on historical data.
- Real-time decision making: Adjusting processes dynamically according to incoming data.
- Autonomous operations: Enabling systems to operate with minimal human intervention.
The Importance of Control System Machine Learning in Business
The application of control system machine learning has transformed various sectors by optimizing performances and driving growth. Here are some compelling reasons why businesses should adopt these technologies:
1. Improved Efficiency and Productivity
Businesses that implement control system machine learning can automate numerous processes, thus increasing productivity. For instance, predictive maintenance systems use historical data to forecast equipment failures, minimizing downtime and running costs.
2. Data-Driven Decision Making
With machine learning, organizations can analyze larger datasets than ever before, uncovering hidden patterns that inform strategic decisions. This capability ensures that decisions are based on quantifiable data, reducing uncertainty and risks.
3. Enhanced Data Governance
Control system machine learning aids in data governance by ensuring that data privacy, security, and compliance measures are effectively implemented. Systems can monitor data access and usage patterns to identify anomalies, making it easier to adhere to regulatory requirements.
4. Tailored Customer Experiences
Businesses can leverage machine learning to analyze customer behavior and preferences, delivering personalized services to enhance satisfaction and loyalty. This capability leads to improved customer retention and higher lifetime value.
Applications of Control System Machine Learning
The versatility of control system machine learning makes it applicable across various domains. The following sections highlight several key industries and their respective applications:
1. Manufacturing
In manufacturing, control system machine learning is used to monitor production processes, optimize supply chain management, and maintain quality control. By analyzing operational data, manufacturers can fine-tune their processes to reduce waste and inefficiencies.
2. Healthcare
In the healthcare sector, machine learning algorithms enhance patient outcomes by analyzing medical data for predictive analytics and disease detection. Control systems in medical devices also use machine learning to ensure optimal functionality and patient safety.
3. Finance
Financial institutions employ control system machine learning to detect fraudulent transactions, assess credit risks, and automate compliance processes. By analyzing transaction patterns, these systems can flag suspicious activities in real-time, greatly enhancing security.
4. Transportation and Logistics
The transportation industry utilizes control system machine learning for route optimization, traffic management, and predictive maintenance of vehicles. These applications help in minimizing operational costs and improving delivery times.
Challenges and Considerations
While control system machine learning holds immense potential for businesses, it is essential to be aware of the challenges involved in its implementation. Some common challenges include:
- Data Quality: Ensuring high-quality data is crucial for effective machine learning outcomes. Poor data quality can lead to inaccurate models and misleading results.
- Integration Complexity: Integrating machine learning models into existing control systems requires careful planning and execution to avoid disruptions.
- Skills Gap: Organizations must invest in training their teams or hiring qualified professionals capable of developing and maintaining machine learning systems.
Best Practices for Implementing Control System Machine Learning
To successfully implement control system machine learning, businesses should consider the following best practices:
- Define Clear Objectives: Establish clear goals for what you want to achieve with machine learning applications.
- Choose the Right Technologies: Invest in robust technologies that align with your business needs and objectives.
- Data Management Strategy: Develop a comprehensive data governance framework to ensure data quality and compliance.
- Iterative Learning: Embrace an agile approach by continually refining algorithms based on new data and insights.
Future Trends in Control System Machine Learning
The future of control system machine learning promises exciting advancements as businesses adapt to the evolving technological landscape. Some anticipated trends include:
- Increased Autonomy: Systems will become increasingly autonomous, leading to reduced human intervention in control processes.
- Enhanced Collaboration: Integration of machine learning with IoT devices will allow for enhanced communication and collaboration across systems.
- Real-Time Analytics: The demand for real-time data processing will drive the development of faster and more efficient machine learning algorithms.
Conclusion
In conclusion, control system machine learning is revolutionizing the way businesses operate by enhancing efficiency, driving data-driven decisions, and improving customer experiences. As organizations continue to recognize the potential of this technology, those that adopt machine learning will not only maintain a competitive edge but also pave the way for innovative practices in their respective industries.
For businesses looking to implement machine learning solutions, exploring platforms like Intalio can provide the necessary tools and expertise to thrive in the digital age. Embracing these technologies can lead to unprecedented success, making your business future-ready.