Unlocking Opportunities: The Role of Healthcare Datasets for Machine Learning
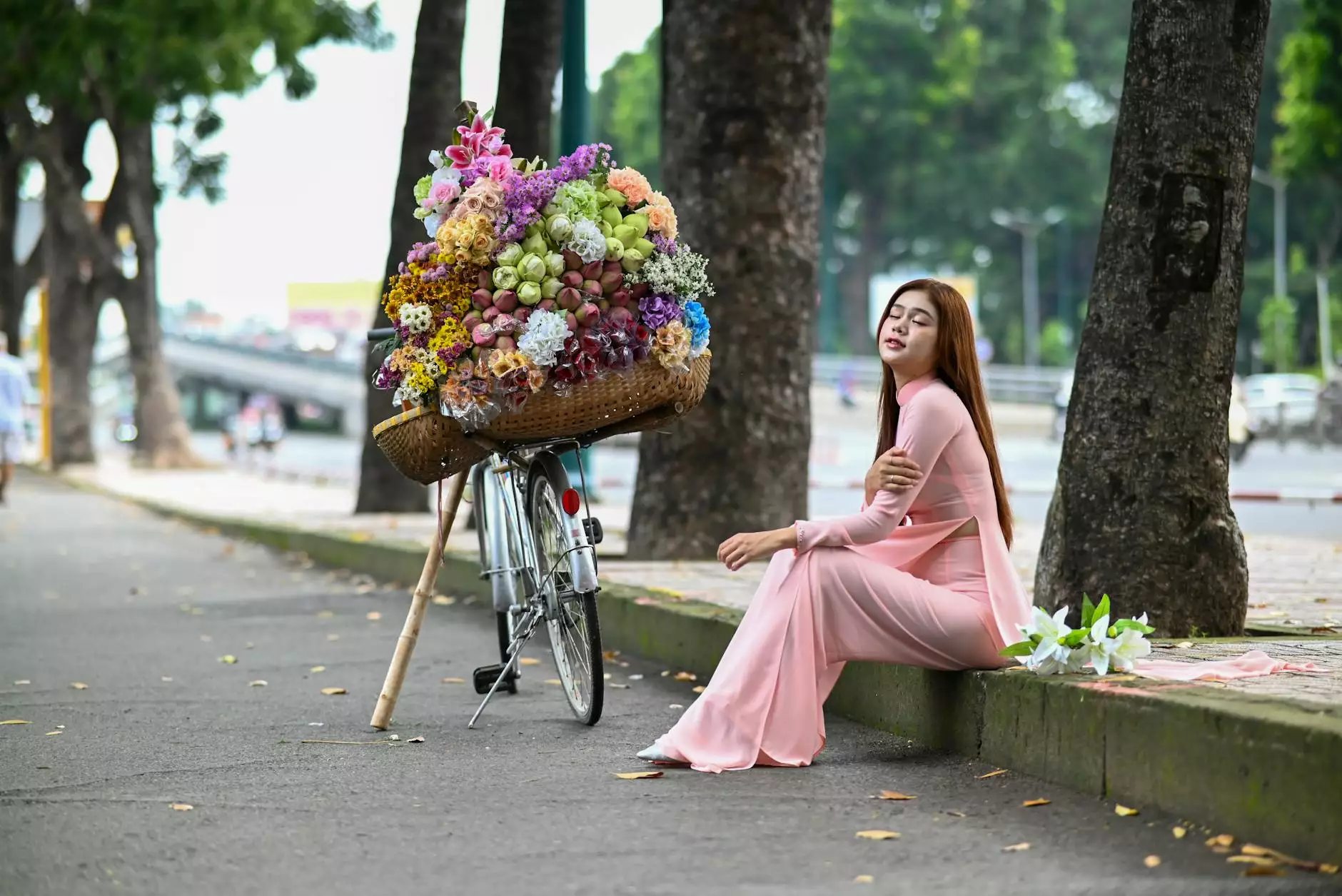
In today's rapidly evolving technological landscape, machine learning (ML) stands as a transformative force across various sectors. One of the most promising areas of application is in healthcare. The integration of healthcare datasets for machine learning holds the potential to not only improve patient outcomes but also enhance operational efficiencies within healthcare organizations. In this comprehensive article, we will delve into the significance of healthcare datasets in the domain of machine learning and explore their implications for businesses, particularly in the fields of Home Services and Keys & Locksmiths.
Understanding Healthcare Datasets
Healthcare datasets are collections of structured and unstructured data derived from various healthcare sources. These datasets encompass a wide range of information, including:
- Patient records: Detailed health histories, treatment plans, and outcomes.
- Clinical trials data: Results and participant information from clinical studies.
- Billing and insurance claims: Financial records associated with patient treatment.
- Wearable device data: Information collected from health monitoring devices.
- Social determinants of health: Factors such as socioeconomic status, education, and environment that impact health outcomes.
The Importance of Datasets for Machine Learning
The nature of healthcare datasets for machine learning is critical because the effectiveness of machine learning algorithms heavily depends on the quality and quantity of data. These datasets enable machines to recognize patterns, make predictions, and automate processes that would otherwise require human intelligence. Here are some key reasons why the availability of healthcare datasets is vital:
- Data-driven decisions: Organizations can leverage insights drawn from data to inform strategic decisions.
- Predictive analytics: Machine learning models can predict patient outcomes and disease patterns, leading to proactive care.
- Personalization of treatments: Datasets allow for more personalized healthcare plans tailored to individual patient needs.
- Operational efficiency: Streamlining processes reduces costs and improves patient satisfaction.
Real-world Applications of Healthcare Datasets
The applications of healthcare datasets for machine learning are extensive, offering solutions to some of the most pressing challenges within the healthcare sector. Some notable real-world applications include:
1. Predictive Modeling for Disease Diagnosis
Machine learning algorithms can analyze past patient data to predict potential diagnoses. For instance, predicting the likelihood of diabetes based on a patient's historical health records can enable early intervention.
2. Treatment Optimization
Datasets can help identify which treatment options are most effective for specific patient demographics, thereby optimizing treatment plans.
3. Patient Monitoring and Management
Wearable devices collect health data that can be analyzed in real time, allowing for continuous monitoring of patients and timely intervention when health metrics indicate a potential issue.
4. Resource Allocation
By analyzing patterns in patient admissions and treatments, machine learning can help healthcare organizations allocate resources more effectively, reducing waiting times and improving patient care.
The Intersection of Healthcare and Business
For businesses, particularly in the Home Services and Keys & Locksmiths categories, integrating insights from healthcare datasets presents unique opportunities. By adopting a data-driven approach, these businesses can enhance service delivery and customer engagement. Here are some ways this intersection can occur:
Enhancing Customer Engagement
Understanding customer health needs through data can help businesses develop targeted marketing strategies that resonate with their audience. For example:
- Tailored Services: Offering services that meet specific healthcare needs of customers, such as improved accessibility features in homes for elderly clients.
- Community Programs: Collaborating with local healthcare providers to offer workshops or services that promote health and well-being.
Innovation in Service Delivery
Data analysis can reveal opportunities for innovation in service delivery, leading to improved customer experiences. Examples include:
- Smart Home Solutions: Incorporating health-monitoring technology into home services, enabling prompt responses to health-related issues.
- Flexible Scheduling: Using algorithms to optimize service schedules based on customer health needs or past service data.
Streamlining Operations
The application of machine learning can also streamline operations within the business. By analyzing data on service requests and customer preferences, businesses can improve logistics and inventory management. For instance:
- Predictive Maintenance: Using data to predict when a locksmith tool may need servicing or replacement based on usage patterns.
- Efficiency in Dispatch: Machine learning can optimize routes for locksmiths and technicians, reducing time spent on the road and increasing service capacity.
Challenges in Utilizing Healthcare Datasets
While the potential benefits of healthcare datasets for machine learning are immense, several challenges must be addressed:
Data Privacy and Security
One of the foremost concerns in utilizing healthcare datasets is ensuring the privacy and security of patient information. Robust data governance practices must be established to protect sensitive data from breaches and misuse.
Data Quality and Standardization
The variability in how data is collected and stored poses a challenge for effective analysis. Ensuring data quality and standardization across datasets is crucial for accurate machine learning outcomes.
Technical Expertise
Implementing machine learning solutions requires technical expertise that may not be available within all organizations. Continual training and collaboration with data scientists are essential for successful integration.
The Future of Healthcare Datasets and Machine Learning
The landscape of healthcare and machine learning is rapidly evolving. As technology advances, the capacity for healthcare datasets for machine learning to transform industries will only grow. The convergence of artificial intelligence (AI), big data, and healthcare is poised to revolutionize how businesses operate and interact with customers.
Emerging Trends to Watch
- Increased Use of AI: We can anticipate greater integration of AI technologies to harness the vast amounts of healthcare data available.
- Telehealth Expansion: As telehealth becomes more prominent, datasets from virtual consultations will play a crucial role in understanding patient outcomes.
- Interoperability Advances: Future advancements in data interoperability will facilitate seamless sharing of healthcare information across platforms.
Conclusion
The potential for healthcare datasets for machine learning to reshape healthcare and business practices is significant. By embracing a data-driven culture, businesses in the Home Services and Keys & Locksmiths domains can harness insights that not only improve customer engagement and operational efficiency but also contribute positively to community health outcomes. As we look to the future, capitalizing on these datasets will be key to thriving in an increasingly competitive market.